You signed in with another tab or window. Reload to refresh your session.You signed out in another tab or window. Reload to refresh your session.You switched accounts on another tab or window. Reload to refresh your session.Dismiss alert
I’m currently running MIN1PIPE on Miniscope videos recorded in the VTA. As VTA is too deep, it has a smaller field of view and a bulb of light covering the centre of the video.
Hence, I used a 24 structural element size and it worked well.
However, when it comes to seeds classification, it identified many seeds for only a few overlapping neurons at the end. (See image attached)
I wonder if you have encountered this before. Is there a way to work around this?
My thoughts are to either train a new RNN for this setting or run CNMF again on the data_processed.m by roifn * sigfn.
Many thanks
The text was updated successfully, but these errors were encountered:
Hi sorry for the really late reply. To solve this problem, first an improved signal to noise ratio will certainly help. Second, I suggest you spatially downsample the video as much as possible so that you can use SE size within the range of [3,7]. The root of this issue, however, is still the low signal level within the FOV, so that the algorithm cannot tell the neurons from the background.
I’m currently running MIN1PIPE on Miniscope videos recorded in the VTA. As VTA is too deep, it has a smaller field of view and a bulb of light covering the centre of the video.
Hence, I used a 24 structural element size and it worked well.
However, when it comes to seeds classification, it identified many seeds for only a few overlapping neurons at the end. (See image attached)
I wonder if you have encountered this before. Is there a way to work around this?
My thoughts are to either train a new RNN for this setting or run CNMF again on the data_processed.m by roifn * sigfn.
Many thanks
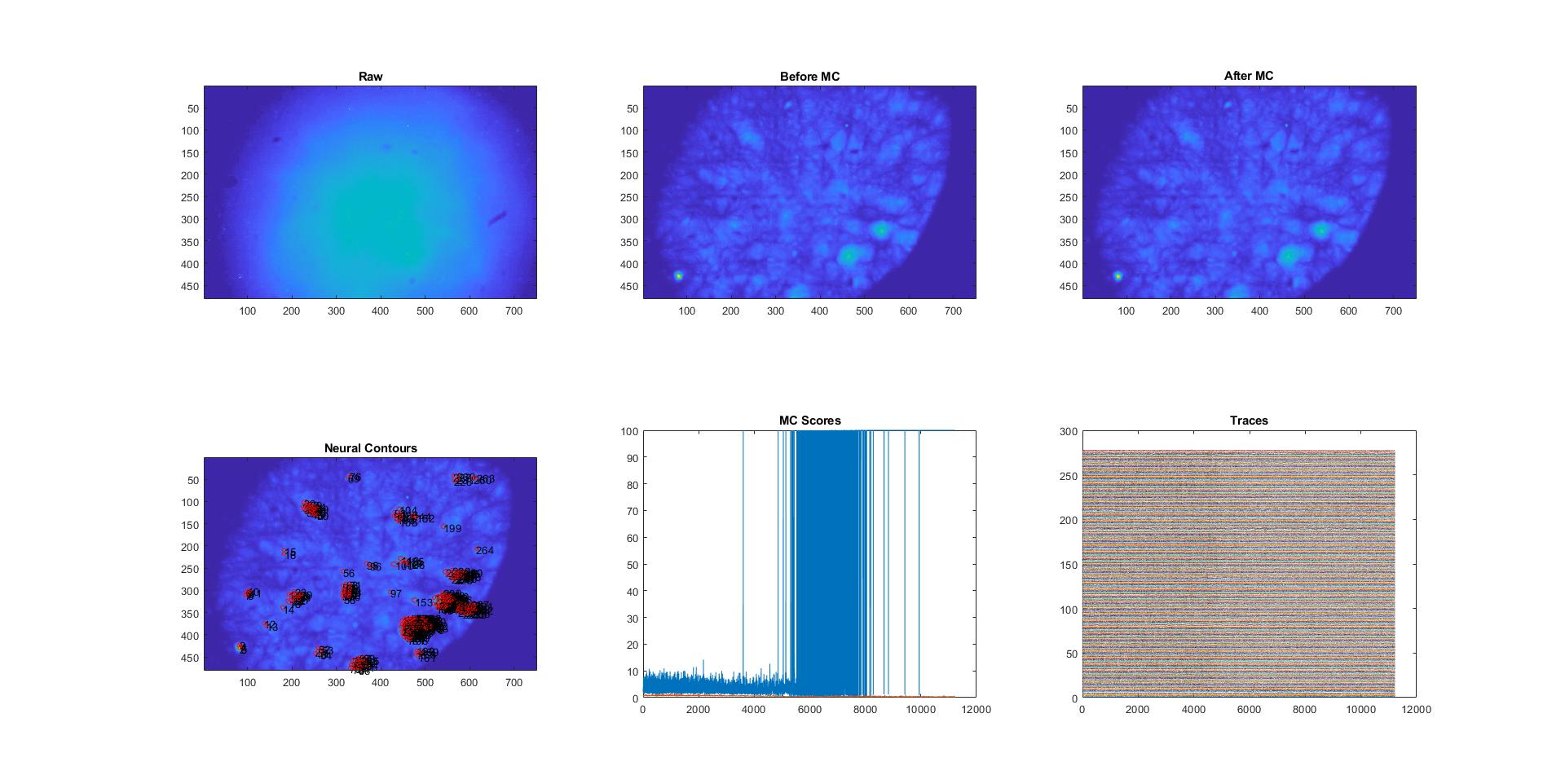
The text was updated successfully, but these errors were encountered: